
AI shown to dramatically speed protein engineering
Study findings promise to shift the field away from trial-and-error approach and toward computational approaches.Media Contact: Leila Gray - 206-475-9809, leilag@uw.edu
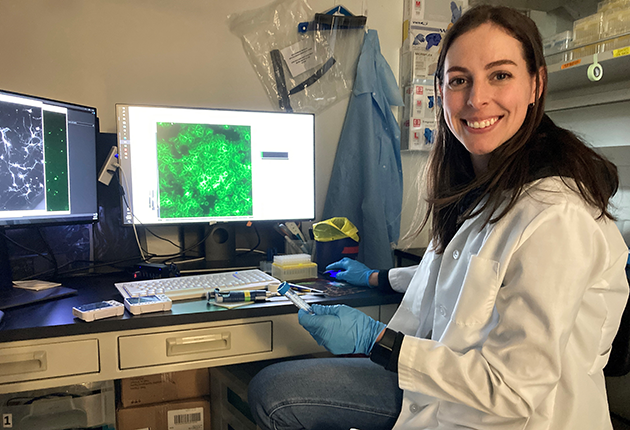
Protein engineering scientists have been able to use machine learning to design proteins that are more efficient at performing a biochemical task. This approach shortened a process that typically takes months to years of trial and error.
Currently, researchers design proteins by introducing mutations into a protein’s amino acid sequence in the hope those mutations will give the protein a desired property or function. They then must repeatedly test the resulting mutant proteins in the lab.
“We were able to circumvent the trial-and-error approach by using machine learning algorithms to predict how different mutations would perform in the real world,” said Sarah Wait, first author of the paper describing the approach. The findings appear in the journal Nature Computational Science.
Wait is a graduate student in the lab of Andre Berndt, assistant professor in the University of Washington Department of Bioengineering and the paper’s senior author.
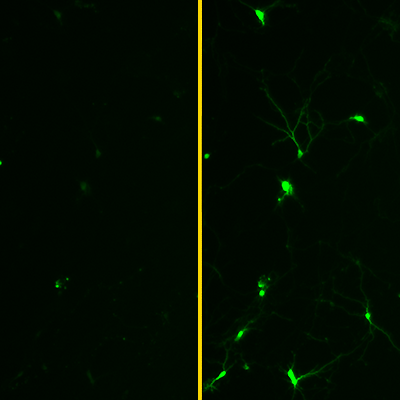
“The impact of this study will be huge,” Berndt said. “It’s going to shift protein engineering away from the hit-and-miss approaches and spur people to invest more into machine learning and other computational approaches.”
In the study, the researchers focused on a protein called GCaMP, which stands for Genetically Encoded Calcium Indicator Modified for Phototransduction. GCaMP — pronounced “G-camp” — fluoresces when it binds to calcium.
This property makes it ideal for studying nerve cell function, because when nerve cells fire, the calcium levels within them rise. When GCaMP is introduced into nerve cells, the cells fluoresce when they fire. This makes it possible to monitor and analyze neuronal activity in real time.
The researchers used a machine learning approach in which algorithms are trained on existing data to create statistical models that can then be used to analyze new data.
In this case, the researchers trained three algorithms on the amino acid sequences of more than 1,000 versions of GCaMP whose properties were known. Some sequences were known to be more efficient and some less. This allowed the algorithms to develop statistical models that could identify sequences that were likely to be more efficient.
Other than the sequences of the different versions of the protein, no other details, such as information about the structure of a protein, were provided to the algorithm.
The researchers combined the results of the three algorithms to increase the chances the results were reliable.
“How each algorithm learned and formulated its prediction was different, so we figured if all three agreed on something there must be something to it,” Wait said.
Then the trained algorithms analyzed the sequences of 1,423 versions of GCaMP whose properties were unknown, and predicted which would likely be the most efficient.
“These were sequences we had never tested, and we didn’t have a clue whether any would be more effective,” Wait said.
The properties they sought in the candidate GCaMP proteins were the ability to fluoresce brightly when exposed to calcium but then quickly dim and be ready to fluoresce again. These properties are ideal because they allow GCamP to more accurately reveal the activity of rapidly firing neurons.
“You want a bigger, brighter signal that quickly turns off,” Wait said.
The machine learning algorithms identified three promising versions of GCaMP. Subsequent laboratory testing found that all three were brighter and faster than any previously reported GCaMP proteins. One variant, called eGCAMP2+, was twice as bright as the versions currently considered state of the art.
The approach can be used to study any protein, not just GCaMP, Wait noted.
“It’s unbiased, meaning it doesn’t care what mutation dataset it sees, so researchers can use this platform to evaluate other proteins of interest,” she said.
In a matter of months, Berndt noted, the algorithms were able to discover significantly improved G-CaMP versions that had taken more than 20 years and a substantial investment in resources to develop.
This work was supported by the National Science Foundation (DGE-2140004), National Institute of Mental Health (RF1MH130391), National Institute of Neurological Disorders and Stroke (U01NS128537), National Institute on Drug Abuse (R21DA051193), National Institute of General Medical Sciences (R01 GM139850-01, P30 DA048736-01-Pilot), Herbold Foundation, La Caixa Foundation, Rafael del Pino Foundation, McKnight Foundation, Brain Foundation, Burroughs Wellcome Fund (CASI 1019469) and Searle Scholars Program SSP-2022-107.
– Written by Michael McCarthy
For details about UW Medicine, please visit http://uwmedicine.org/about.